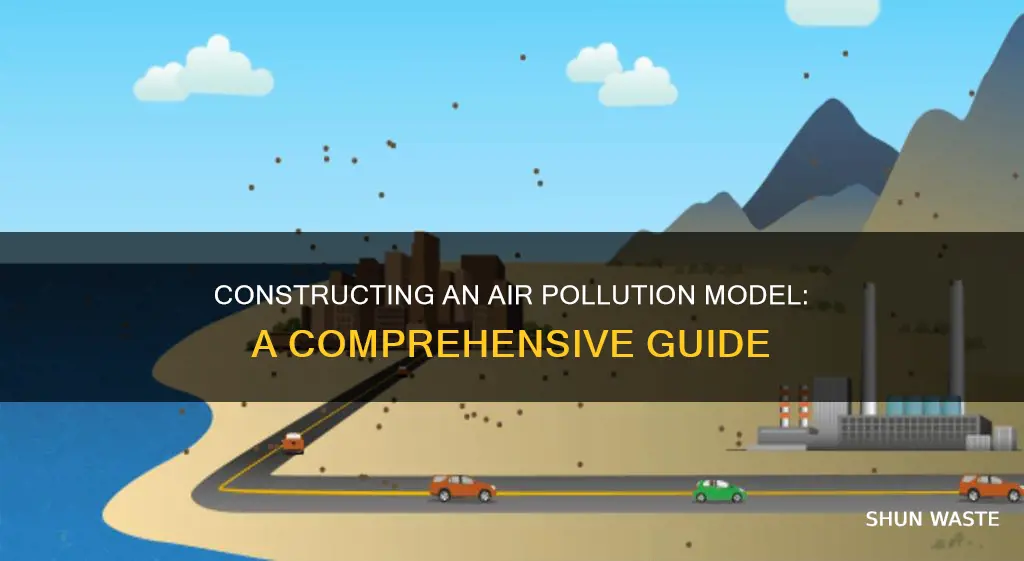
Air pollution modelling is a complex and interdisciplinary scientific area that involves using mathematical theory to understand, predict, and regulate the behaviour of pollutants in the atmosphere. The process involves creating physical or digital models to simulate the spread of harmful airborne material. These models can be used to run hypothetical scenarios, test theories, and understand the environmental impact of different emission rates, weather conditions, and development scenarios. They are also used to assess the impact of air pollutants on human health and the environment. Air pollution modelling is a valuable tool for decision-makers in environmental policy and urban planning, as it provides essential information for improving air quality and mitigating the effects of pollution.
Characteristics | Values |
---|---|
Purpose | To understand, predict, and assess the way pollutants behave in the atmosphere, including their impact on human health and the environment |
Data | Field study data, emission factors, emission rates, meteorological statistics, satellite data, etc. |
Techniques | Mathematical theory, interpolation, Land Use Regression (LUR) models, scaled physical models, etc. |
Scale | Local, regional, or mesoscale |
Timeframe | Short-term (hourly) or long-term (yearly) |
Applications | Regulatory, decision-making, emergency planning, recovery, environmental impact assessment, etc. |
Limitations | Cost, weather conditions, uncertainty due to deficiencies in knowledge of emissions and atmospheric processes |
What You'll Learn
- Using wind tunnels to study airflow and pollution dispersion
- Mathematical theory to predict the behaviour of pollutants
- Land Use Regression (LUR) models to predict air quality
- Remote sensing and data assimilation techniques
- Meteorological statistics and calculation of percentiles and exceedance frequencies
Using wind tunnels to study airflow and pollution dispersion
Wind tunnels are an effective method for studying airflow and pollution dispersion. They are used to develop and test air pollution models, which refer to the use of atmospheric models to understand, assess, and regulate the distribution of toxic pollutants in the atmosphere. This includes their impact on human health and the environment.
Wind tunnels allow researchers to study airflow and the dispersion of pollutants in a controlled environment. They can mimic different meteorological conditions, terrain, and topographical features, making it easier to control parameters such as velocity and turbulence intensity. This enables researchers to obtain useful data that can be translated into real-life situations. For example, researchers can study the impact of building shapes, sizes, and their orientation to streets on the quantity and direction of pollutants in the wind flow.
One of the advantages of using wind tunnels is the ability to produce a set of physical data. This data can then be used to validate computational models, such as Computational Fluid Dynamics (CFD) models, which are used to predict airflow and the dispersion of pollutants. By comparing the results of wind tunnel experiments with CFD simulations, researchers can improve the accuracy of their models.
Additionally, wind tunnels can be used to study the impact of different emission rates and sources on air pollution. For instance, the effects of wind direction and speed on the dispersion of pollutants can be examined. This information can be used to inform decision-making on air quality issues and evaluate mitigation options.
Overall, wind tunnels play a crucial role in studying airflow and pollution dispersion, providing valuable insights that can be used to improve air quality models and better understand the complex nature of air pollution.
Air Pollutants: Understanding the Invisible Danger Around Us
You may want to see also
Mathematical theory to predict the behaviour of pollutants
Air pollution modelling uses mathematical theory to predict the behaviour of pollutants in the atmosphere. This involves understanding, assessing, and regulating the distribution of toxic pollutants, including their impact on human health and the environment.
Mathematical modelling is a practical method to relate source emissions to air pollution concentrations. It simulates pollutant evolution by interrelating symbolic descriptions of the physical and chemical processes occurring in the atmosphere within a computational framework. The multistep process begins with characterising the emissions, then determining the effects that atmospheric transport and chemical reactions have on pollutant concentrations. This is followed by correlating people's activities with pollutant concentrations to determine personal exposure, which is related to dose, and finally, the health effects.
Mathematical models are based on assumptions, rules, and data. For instance, the assumption that the atmosphere behaves predictably and that plumes behave in a known way. Rules include emission factors and emission rates, such as the volume and composition of an emission source, and the reactions emissions undergo in the atmosphere.
Interpolation is a popular method for large data sets as it can break data into smaller subintervals that can be manipulated to fit the user's purpose. Another method is Land Use Regression (LUR), which predicts air quality based on existing knowledge of air quality and land use in a given location.
Mathematical models can be used to predict dangerous pollution levels and provide recommendations to avoid undesirable situations. They can also be used to assess hypothetical situations, such as predicting the emissions from a proposed industrial facility and their impact on the surrounding area.
Nitrous Oxide: Air Pollutant or Not?
You may want to see also
Land Use Regression (LUR) models to predict air quality
Land Use Regression (LUR) models are a popular method for predicting air quality in densely populated areas. LUR models are based on predictable pollution patterns and use multiple regression equations to estimate pollution concentrations in a given area. Geographic information systems (GIS) are often used to collect measurements of environmental variables such as traffic, topography, and other geographic factors.
The LUR model can be used to predict pollution concentrations at unmeasured locations by establishing an equation that relates sample locations to these environmental variables. This approach has been applied in several cities, including London, New York, Toronto, Amsterdam, Prague, and Stockholm. The model is particularly useful for screening studies and can substitute for dispersion models when there is insufficient data.
The accuracy of LUR models can be improved by adding prediction variables such as holiday traffic variants and seasonal meteorological variations. The incorporation of Geographically Weighted Regression (GWR) can also enhance the model's explanatory power by reducing the effects of spatial non-stationarity, which occurs when variables form inconsistent relationships over large areas.
LUR models have been used to predict the concentration of various pollutants, including nitrogen dioxide (NO2), ultrafine particles, lung-deposited surface areas, and markers of particulate matter pollution. For example, in the Swiss SAPALDIA study, LUR models were developed for biannual average concentrations of PM2.5, PM10, PNC, and LDSA. The availability of common-source predictor data is crucial for the successful transfer and combination of LUR models across different areas.
Overall, LUR models are a valuable tool for predicting air quality, particularly in urban settings. By utilizing geographic and environmental data, LUR models can estimate pollution concentrations and provide insights into the health effects of air pollution on individuals residing in these areas.
Airplanes' Impact: Polluting Our Skies and Atmosphere
You may want to see also
Remote sensing and data assimilation techniques
Data assimilation, on the other hand, involves the collection and interpretation of data from various sources, such as field studies, wind tunnel tests, and computer simulations, to improve the accuracy of air pollution models. Field studies provide information about emissions and local air quality, while wind tunnel tests help understand the dispersion of pollutants and their impact on public health. Data assimilation techniques also include mathematical and computational methods to process and analyse large and complex datasets efficiently.
One example of data assimilation is the use of Land Use Regression (LUR) models, which predict air quality by analysing the relationship between air quality data and factors such as vehicle movements, street size, and building height. Another technique is interpolation, which is useful for large and sparse datasets as it breaks the data into smaller subintervals that can be manipulated to fit specific purposes.
Air Pollution: Worsening COVID-19, A Lethal Combination
You may want to see also
Meteorological statistics and calculation of percentiles and exceedance frequencies
Air pollution modelling is a complex and interdisciplinary scientific area that involves understanding, assessing, and regulating the distribution of toxic pollutants in the atmosphere and their impact on human health and the environment. This process is closely related to meteorological fields as the atmosphere is governed by general fluid mechanics laws described by the Navier-Stokes equation system.
Meteorological statistics are crucial for constructing an air pollution model, particularly when dealing with urban-scale problems like local circulation systems and sea and land breezes. These statistics are necessary for calculating percentiles and exceedance frequencies. For example, in the case of uniform terrain, representative meteorological data, along with appropriate emission data, can be used in Gaussian models to provide reliable long-term average values for inert pollutants such as SO2, NOX, and lead.
Exceedance frequencies refer to the frequency with which certain thresholds are exceeded. In the context of air pollution modelling, these thresholds could be related to pollutant concentrations, weather conditions, or other relevant factors. For instance, an analysis might show that under specific wind conditions, pollutant concentrations in a residential area exceed acceptable levels.
Calculating percentiles and exceedance frequencies is essential for understanding the distribution and impact of pollutants. Percentiles help identify the percentage of time that pollutant concentrations fall within a certain range, while exceedance frequencies indicate how often those thresholds are surpassed. These calculations can be done using time series data, with models providing outputs like annual averages and percentile values.
To ensure the accuracy of air pollution models, it is crucial to collect detailed meteorological and emission data. Specialists are often required when dealing with sophisticated models or areas with complex meteorological or topographic features. By combining field study data with controlled experiments in wind tunnels, researchers can refine their models and improve their understanding of air pollution behaviour and its impact on public health.
Bicycles: Reducing Air Pollution, Improving Health
You may want to see also
Frequently asked questions
Air pollution modelling is the development and use of atmospheric models to understand, assess, and regulate the distribution of toxic pollutants in the atmosphere, including their impact on human health and the environment.
Air pollution modelling allows us to assess a hypothetical situation before it occurs. For example, we can predict the emissions from a new industrial facility and their impact on the surrounding area. This allows us to solve potential problems before they occur.
There are a variety of methods and techniques used, including mathematical theory, meteorological statistics, remote sensing, land-use impact, data assimilation techniques, and chemical schemes. The choice of method depends on the specific application and goals of the model.
Air pollution models use data on emissions, atmospheric processes, and dispersion patterns to make predictions about air quality. This data can come from field studies, wind tunnel tests, satellite observations, and emission inventories.
It is important to understand the strengths and weaknesses of your model. You can validate your model by comparing the results with measurements or combining information from modelling and measurements.