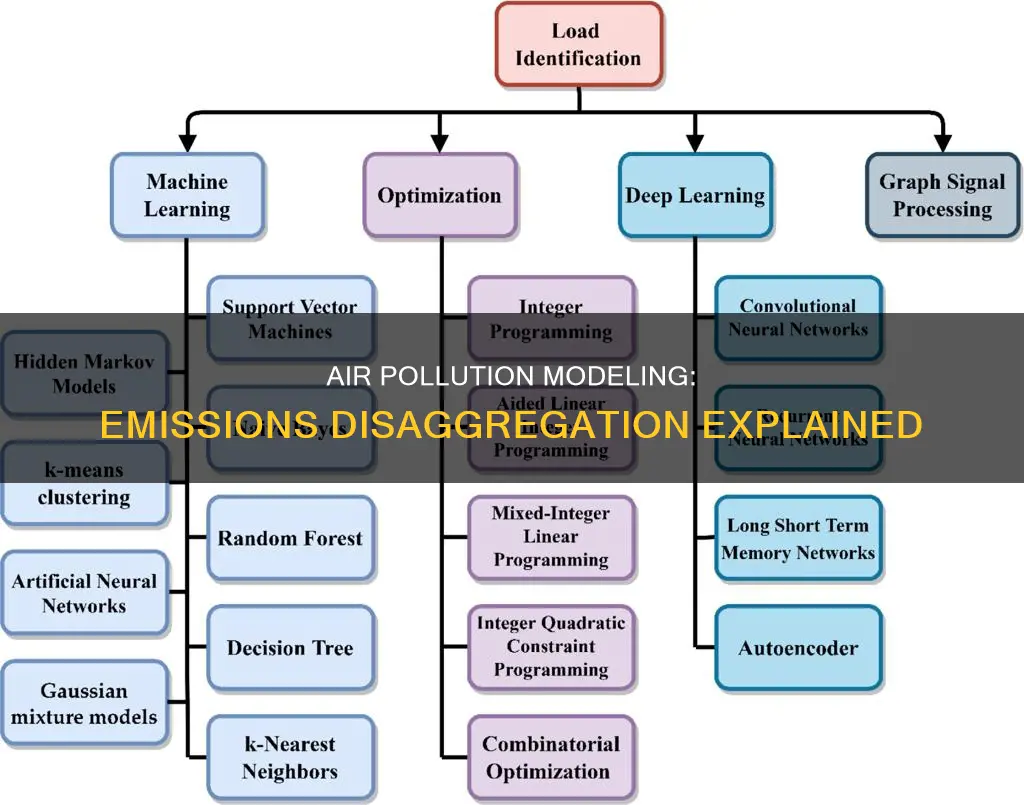
Air pollution modelling is a critical tool for understanding the relationship between sources of pollution and their effects on air quality. It involves the use of various models and simulations to estimate and predict the impacts of emission sources on ambient air quality. This process is known as emissions disaggregation air pollution modelling. This type of modelling is essential for guiding air quality forecasting and mitigation efforts, particularly in urban areas where ground transportation is a major contributor to air pollution. By combining real-world measurements with laboratory data, modellers can quantify and spatially disaggregate emissions data, providing valuable insights for developing emission reduction strategies and informing policy decisions.
Characteristics | Values |
---|---|
Purpose | To provide information on air quality, the relation between air concentrations and emissions, and to support air quality management. |
Data Sources | Real-world measurements, laboratory data, meteorological data, emission inventories, air quality data. |
Models | Bottom-up (microscale), top-down (macroscale), Gaussian, CMAQ, AERMOD, DROVE, MOVES, IVE, COPERT, etc. |
Applications | Predicting impacts of potential emission sources, simulating ambient pollution concentrations, determining relative contributions of sources, monitoring compliance with regulations, policy decisions, control strategies, emission regulations, mitigation options, etc. |
Limitations | Modelled information is uncertain due to deficiencies in knowledge of emissions and atmospheric processes; spatial and temporal averaging complicates direct comparisons with measurements. |
What You'll Learn
Ground transportation emissions
Ground transportation, including the road and rail sectors, is a major source of greenhouse gas (GHG) emissions. To model ground transportation emissions, emission modelers should combine real-world measurements with laboratory data. This involves quantifying emissions of carbon monoxide, nitrogen oxides, sulfur dioxide, and particulate matter (PM2.5) and then disaggregating these emissions spatially and temporally.
There are two principal approaches to compiling emission inventories: the bottom-up (microscale) and top-down (macroscale) approaches. The bottom-up approach is more accurate but expensive and time-consuming, while the top-down approach is faster, cheaper, and requires fewer technical skills, but may be less accurate. Emission inventories can be used as input data for pollutant dispersion models and air quality forecasts.
When modelling ground transportation emissions, it is important to consider the local circulation systems, such as sea and land breezes, which may impact the dispersion of pollutants. Meteorological statistics, including wind conditions, are needed to calculate percentiles and/or exceedance frequencies. Additionally, the short distances between roads and buildings can impact indoor air quality.
To address the impact of ground transportation emissions, policies such as emissions trading systems (ETS) have been proposed to control carbon emissions from fossil fuel combustion during transport activities. However, existing upstream policies have been found to lack stringency and effectiveness, while downstream policy implementation remains limited. As such, a downstream ground transportation emissions trading system (GTETS) that incorporates new technologies has been proposed.
Overall, modelling ground transportation emissions involves quantifying and disaggregating emissions, utilizing emission inventories, considering local circulation and meteorological factors, and implementing policies to mitigate the environmental and health impacts of these emissions.
Asthma and Air Pollution: What's the Main Culprit?
You may want to see also
Bottom-up and top-down approaches
Air pollution modelling is a method for providing information on air quality based on emissions and the atmospheric processes that lead to pollutant dispersion. To assess emissions from various sources, two different approaches are often considered: the “top-down” and the “bottom-up” approaches.
The "top-down" approach starts from values of annual emissions assessed at the national level and is then disaggregated at different levels, such as the provincial and municipal levels, using statistical indicators like population, roads, and land use. This methodology also allows for temporal disaggregation, providing hourly emission resolutions from annual data. This approach is faster, cheaper, and requires fewer technical skills from developers, but may be less accurate.
On the other hand, the "bottom-up" approach begins with local data at the municipal level or even from specific emission sources, such as road graphs or industry locations. Using this information and proper emission factors, the approach assesses hourly emissions directly at the local level. The bottom-up method is generally more accurate but tends to be more expensive and time-consuming.
Both approaches are useful for different reasons and are often used in combination or in intermediate forms. For example, in the case of a study on traffic emissions in Manizales, Colombia, the bottom-up approach showed higher emissions for pollutants such as NMVOC, PM10, and CO, while the top-down approach showed higher emissions for NOX. In another instance, a study in the Ravenna Province in Italy used three top-down models to disaggregate emissions from a provincial to a local scale, with the model combining resident population and building volume performing the best.
The choice between these approaches depends on the specific context and goals of the emissions disaggregation modelling. It is important to consider the advantages and disadvantages of each method, as well as the availability of data and the desired level of accuracy.
Air Pollution's Impact on Animal Habitats and Health
You may want to see also
Air quality modelling
Emissions inventories are essential for guiding air quality forecasting and mitigation efforts. These inventories are compiled through two principal approaches: bottom-up (microscale) and top-down (macroscale). The bottom-up approach is more accurate but expensive and time-consuming, while the top-down approach is faster, cheaper, and requires fewer technical skills. The top-down approach is particularly useful for understanding domestic heating emissions.
The availability of appropriate data should be investigated before applying any model. Simple models may be appropriate for first screening purposes, or in cases of limited input information. More sophisticated models may be required for complicated meteorological or topographic features. Models such as DROVE (an algorithm for spatial and temporal disaggregation of on-road vehicle emission inventories) can be implemented worldwide and provide valuable information for developing emission reduction strategies.
Air quality dispersion models predict the impact of pollutants released from various sources such as power plants and roadways. The Air Quality Dispersion Model (AERMOD) is the US EPA's preferred dispersion modelling system. It is used to model the impact on air quality from sources that emit a variety of pollutants, including carbon monoxide, lead, sulfur dioxide, nitrogen dioxide, and hazardous air pollutants.
Photochemical modelling is another technique used in air quality modelling. It involves preparing emissions for input into a photochemical model. In most cases, emissions are not measured directly but are estimated using a variety of models.
Air Pollutants: Understanding Common Toxins in the Air
You may want to see also
Pollutant dispersion
The dispersion models consider various factors, including emissions data, meteorological conditions, and atmospheric processes, to predict the concentration of pollutants at specific downwind locations. This helps determine compliance with air quality standards and regulations, such as the National Ambient Air Quality Standards (NAAQS) in the United States.
There are different types of dispersion models, including the box model, which assumes a box-shaped air shed with homogeneously distributed pollution, and the Lagrangian model, which calculates pollution dispersion by tracking the trajectories of pollution plume parcels. Modern dispersion modelling programs often include pre-processor modules for inputting meteorological data and post-processor modules for graphing output data or mapping impacted areas.
The choice of model depends on the availability of detailed input data and the complexity of meteorological or topographic features. Simple models may be suitable for screening purposes or when input information is limited. However, more sophisticated models may require specialist involvement.
Air Pollution: Harmful Effects on Human Health Explained
You may want to see also
Meteorological data
Meteorological modelling techniques are employed to simulate and predict meteorological conditions. These models utilise universal laws of atmospheric physics and empirical relationships to calculate various parameters. For instance, the Texas Commission on Environmental Quality (TCEQ) employs state-of-the-art four-dimensional computer models that incorporate atmospheric physical laws and measured observations to forecast weather conditions in both time and space. The basic structure of a meteorological model involves using these laws and relationships to calculate wind flow, temperature, humidity, vertical air mixing, and other parameters within three-dimensional grid boxes covering the domain of interest.
Furthermore, meteorological data plays a vital role in understanding the health impacts of air pollution. By integrating meteorological data with emission data, researchers can assess the potential human exposure and long-term repercussions on population health. Meteorological variables, such as wind speed and solar radiation, influence the dispersion and concentration of air pollutants, thereby impacting public health. Overall, meteorological data is indispensable for comprehensive emissions disaggregation and air pollution modelling, enabling scientists to make informed decisions and develop effective strategies to mitigate air pollution and safeguard public health.
Construction's Air Pollution: Understanding the Haze and Dust
You may want to see also
Frequently asked questions
Emissions disaggregation air pollution modeling is a method for providing information on air quality based on our understanding of emissions and the atmospheric processes that lead to pollutant dispersion, transport, chemical conversion, and removal from the atmosphere.
Emissions data is usually obtained from emission inventories, which are compiled through two principal approaches: bottom-up (microscale) and top-down (macroscale). The bottom-up approach is more accurate but expensive and time-consuming, while the top-down approach is faster, cheaper, and requires fewer technical skills.
Some examples of emission disaggregation models include the EPA's MOVES model, the Canadian National Pollutant Release Inventory, and the Air Quality Dispersion Model (AERMOD).
Air pollution models enable users to estimate the relationship between sources of pollution and their effects on air quality, predict the impacts of potential emission sources, and simulate ambient pollution concentrations under different policy scenarios. They are also critical for monitoring compliance with air quality regulations and making policy decisions.
Emissions disaggregation air pollution modeling has been used in various studies, such as quantifying air pollution emissions from ground transportation in developing countries, analyzing the impact of roadway design on air pollutant exposure, and improving air quality plans in urban areas experiencing rapid urbanization.