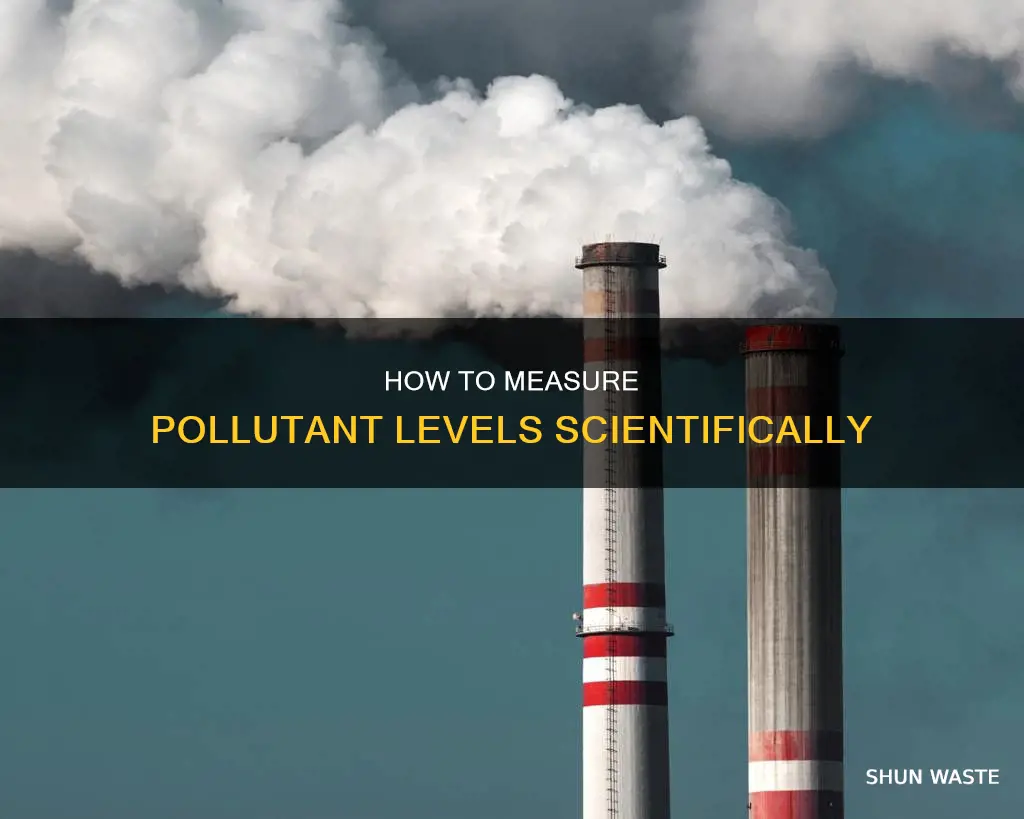
Levels of measurement, also called scales of measurement, refer to the process of categorizing data based on its characteristics and properties. There are four levels of measurement: nominal, ordinal, interval, and ratio. Each level has a different impact on how data can be analyzed. For example, nominal data can be categorized, but not ranked, while ordinal data can be both categorized and ranked. The level of measurement used depends on the nature of the variable and the level of precision required. When discussing levels of pollutants, it is important to determine whether the data is interval or not, as this distinction influences the types of statistical analyses that can be performed.
Characteristics | Values |
---|---|
Levels of Measurement | Nominal, Ordinal, Interval, Ratio |
Interval Variable | Data can be categorized, ranked, and evenly spaced |
Interval Variable vs Ordinal Variable | Ordinal variables are ranked but the intervals between the values are not equally spaced |
Interval Variable vs Ratio Variable | Ratio variables have all the properties of an interval variable, but also have a clear definition of zero |
What You'll Learn
Interval variables are quantitative
Levels of measurement, or scales of measurement, refer to how precisely a variable is measured. In scientific research, a variable is anything that can take on different values across a dataset. For example, height or test scores. There are four levels of measurement: nominal, ordinal, interval, and ratio.
Interval variables are different from nominal variables, which are categorical and cannot be ranked. For example, gender and ethnicity are nominal variables because they cannot be ranked. They can only be categorized. Another example of a nominal variable is hair colour because there is no agreed-upon way to rank hair colours from highest to lowest.
Ordinal variables are also categorical, but they can be ranked. For example, income levels can be ranked from low to high. However, the differences between the intervals may not be equal. For example, the difference between the income levels "less than 50K" and "50K-100K" is not the same as the difference between "50K-100K" and "over 100K".
Ratio variables are the most sophisticated level of measurement. They have all the properties of interval variables but also have a clear definition of zero, which represents a total absence of the variable. For example, weight in kilograms is a ratio variable because something that weighs zero kilograms truly weighs nothing.
The level of measurement used depends on the variable being studied and the research question. In many cases, variables can be measured at different levels, so the level of measurement must be chosen before data collection begins. The level of measurement will determine how the data can be analyzed. Interval and ratio data lend themselves to more complex statistical analysis than nominal and ordinal data.
Ground Pollution: Understanding the Soil Contamination Crisis
You may want to see also
They are numerical and ordered
Levels of measurement describe the characteristics of a variable. There are four levels of measurement: nominal, ordinal, interval, and ratio. These levels are ranked from low to high in terms of complexity and precision.
Interval data is numerical and ordered. It is similar to ordinal data, but the intervals between the values of the variable are equal. For example, temperature in Fahrenheit is interval data because the difference between 10 and 20 degrees Fahrenheit is the same as the difference between 50 and 60 degrees Fahrenheit. Other examples of interval data include standardized tests, such as the SAT, and psychological inventories.
Interval data is quantitative, meaning that it can be measured and expressed numerically. It is also ordered, meaning that there is a known, consistent order to the data. For example, a score of 500 on the SAT is higher than a score of 400.
Interval data is often used in statistics and data analysis. It allows for more complex statistical analysis than nominal or ordinal data. This is because interval data has equal intervals between values, which allows for meaningful comparisons and calculations. For example, the difference between two scores on a standardized test can be interpreted as a meaningful difference in performance.
Interval data does not have a true zero, which distinguishes it from ratio data. On an interval scale, zero is an arbitrary point and does not represent a total absence of the variable. For example, a temperature of zero degrees Fahrenheit does not mean there is "no temperature," it simply means it is extremely cold.
Leaf Blowers: Legal, but Are They Noise Polluters?
You may want to see also
They have equal intervals between values
The levels of measurement, also called scales of measurement, indicate how precisely a variable has been measured. There are four levels of measurement: nominal, ordinal, interval, and ratio. The level at which a variable is measured determines how it can be analyzed.
Interval variables are quantitative and have equal intervals between values. This means that the distances between values are consistent across the scale. For example, the difference between 10 and 20 degrees Fahrenheit is exactly the same as the difference between 50 and 60 degrees Fahrenheit. In this case, zero is an arbitrary point and does not indicate a total absence of the variable.
The levels of measurement are hierarchical, with nominal being the lowest and ratio being the highest. This means that the higher the level, the more complex the measurement. Nominal variables are categorical and have no intrinsic ordering to the categories. Ordinal variables are also categorical but have a clear hierarchy or order to the categories.
Interval variables are similar to ordinal variables in that they both have order. However, interval variables have the added property of equal intervals between values. This means that the differences between values are consistent and meaningful. For example, in a Likert scale, there is an equal distance between each value, such as "strongly agree", "agree", "neutral", "disagree", and "strongly disagree".
In the context of pollutant levels, if we assume that pollutant levels are interval variables, this would imply that there are equal intervals between the different levels of pollutants. For example, if we measure pollutant levels on a scale of 1 to 5, with 1 being the lowest level of pollutants and 5 being the highest, the intervals between each level would be equal. So, the difference between levels 1 and 2 would be the same as the difference between levels 2 and 3, and so on. This would allow for more precise analysis and comparisons between different levels of pollutants.
The Mystery of Lead: Primary or Secondary Pollutant?
You may want to see also
Interval variables are more sophisticated than ordinal variables
Levels of measurement, or scales of measurement, describe the characteristics of a variable and how precisely variables are recorded. In scientific research, a variable is anything that can take on different values across a data set, such as height or test scores.
There are four levels of measurement: nominal, ordinal, interval, and ratio. Nominal data can only be categorized, while ordinal data can be categorized and ranked. Interval data can be categorized, ranked, and evenly spaced, and ratio data can be categorized, ranked, evenly spaced, and has a natural zero. The level of measurement of a variable determines how data can be analyzed. For example, some variables have fixed levels, such as gender and ethnicity, which are always nominal data because they cannot be ranked.
The choice of measurement level depends on the specific context and the nature of the data. For instance, in a psychological study of perception, color would be considered a nominal variable, while in a physics study, color is quantified by wavelength and would be considered a ratio variable. Similarly, income level can be measured using an ordinal scale with categories or a ratio scale with actual income values. Generally, it is preferable to use a ratio scale whenever possible, as it allows for more ways to analyze data.
Green Revolution: Solving Pollution for a Better Tomorrow
You may want to see also
They are used in more complex statistical analysis
The levels of measurement of a variable, also known as its scale or measure, are important distinctions to make when conducting statistical analysis. There are four levels of measurement: nominal, ordinal, interval, and ratio. These levels are hierarchical, with nominal being the lowest and ratio being the highest. The higher the level of measurement, the more complex and precise the analysis can be.
Interval variables are quantitative, meaning they can be used in more complex statistical analyses than nominal or ordinal variables, which are categorical. Categorical variables can only be described, whereas quantitative variables can be measured.
Interval variables are numerical scales that label and order variables, with a known, evenly spaced interval between each of the values. This means that the difference between two values is meaningful. For example, temperature in Fahrenheit is an interval scale because the difference between 10 and 20 degrees Fahrenheit is exactly the same as the difference between 50 and 60 degrees Fahrenheit.
Interval variables are often used in parametric tests to test hypotheses. For example, when conducting a t-test or ANOVA, the assumption is that the distribution of sample means is normally distributed. This can be guaranteed if the distribution of individual observations is normal, or if the sample size is about 30 or larger due to the central limit theorem. Regression analysis assumes that the residuals are normally distributed.
In summary, interval variables are used in more complex statistical analyses because they are quantitative and have meaningful intervals between values. This allows for a wider range of statistical tests to be performed and enables more precise and accurate analyses.
Toxic Pollutants: Understanding Harmful Substances and Their Impact
You may want to see also
Frequently asked questions
An interval variable is a variable that can be categorized, ranked, and has evenly spaced values. Common examples of interval scales include standardized tests, such as the SAT, and psychological inventories.
A ratio variable has all the properties of an interval variable, but with a clear definition of zero. For example, weight in kilograms is a ratio variable because if something weighs zero kilograms, it truly weighs nothing. On the other hand, an interval scale, such as temperature in Fahrenheit, does not have a true zero because a value of zero degrees does not mean there is "no temperature," but rather that it is extremely cold.
An ordinal variable is similar to an interval variable, except that the intervals between the values of the numerical variable are not equally spaced. For example, the difference in educational experience between elementary school and high school is probably much bigger than the difference between high school and some college.
A nominal variable is a variable that can only be categorized, with no meaningful order between the categories. For example, hair color is a nominal variable because there is no agreed-upon way to order the categories (blonde, brown, brunette, red, etc.) from highest to lowest. In contrast, an interval variable has a known, evenly spaced interval between each of its values.