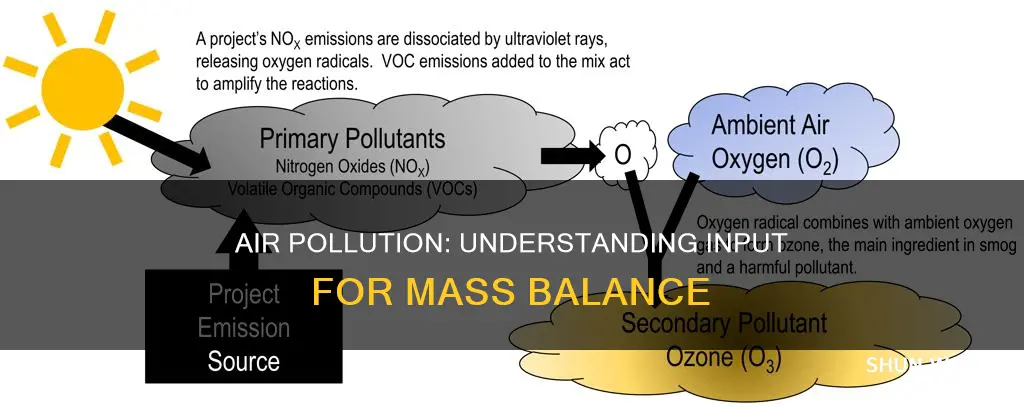
Mass balance is a widely used concept in engineering and environmental analyses. It is used to design chemical reactors, analyse alternative processes to produce chemicals, and model pollution dispersion in physical systems. In the context of air pollution, mass balance models are used to identify and quantify the sources of air pollutants and predict their concentrations. This is particularly important for indoor air quality, as people spend a significant amount of time indoors and pollutants can be more concentrated indoors than outdoors, negatively impacting human health and productivity. Mathematical models, such as the Chemical Mass Balance (CMB) model developed by the EPA, are used to estimate the concentrations of gaseous indoor air pollutants like CO2 and guide necessary arrangements to improve indoor air quality. Mass balance principles can also be combined with machine learning techniques to leverage ambient air quality data and predict air pollution exposure levels within vehicles.
What You'll Learn
Mathematical modelling of indoor air quality
Mass balance is a widely used concept in engineering and environmental analyses. It involves comparing input and output quantities in a system to determine how mass is distributed within it. This principle is applied in the context of air pollution to identify and quantify the sources of pollutants and to model their dispersion.
Mathematical models have been developed to predict CO2 concentrations under different indoor conditions. These models take into account factors such as the number of occupants, room size, ventilation rates, and other relevant parameters. By simulating various scenarios, these models help in understanding the impact of CO2 levels on indoor air quality and guide the design of adequate ventilation systems.
Additionally, mathematical modelling is employed to address particle air pollution, which is another critical aspect of IAQ. Models are used to predict the concentration and fate of particulate matter, considering factors such as ventilation, filtration, deposition onto surfaces, direct emission, and coagulation. These models provide valuable insights into the behaviour and transformation of airborne particles, allowing for the development of effective strategies to mitigate indoor particle pollution.
Furthermore, mathematical models have been developed specifically to assess indoor air quality in relation to smoking activity. These models predict indoor pollutant concentrations from cigarette smoke by applying the laws of conservation of mass. They consider factors such as the number of cigarettes smoked, the volume of the room, and the air exchange rate to estimate respirable particulate concentrations. These models are essential for regulatory bodies and risk assessors to quantitatively evaluate exposure levels and implement appropriate measures to ensure healthy indoor environments.
Industrial Emissions: Air Pollution's Main Culprit
You may want to see also
Sources of air pollutants
Stationary sources, such as power plants, oil refineries, and industrial facilities, emit large amounts of pollution from a single location. These sources contribute to the emission of pollutants like sulfur dioxide, nitrogen oxides, and greenhouse gases. Regulations and retirement of coal-powered plants have helped reduce emissions and associated health risks.
Area sources consist of smaller pollution sources that collectively contribute to air pollution. This includes agricultural areas, cities, and wood-burning fireplaces. Residential wood burning, in particular, has been increasing and accounts for a significant portion of fine particle emissions in some regions.
Natural sources, such as wind-blown dust, wildfires, and volcanoes, can also contribute to air pollution. While they may not always create ongoing pollution problems, natural sources can have significant impacts, especially when pollutants are transported over long distances.
Other sources of air pollutants include industrial processes such as iron, steel, and rubber manufacturing, as well as power generation, which can produce polycyclic aromatic hydrocarbons (PAHs) and volatile organic compounds (VOCs). Vehicle emissions from older diesel engines and the combustion of fossil fuels further contribute to air pollution, emphasizing the importance of regulations and advancements in fuel and engine technology to reduce harmful emissions.
Japan's Air Pollution: Strategies and Solutions
You may want to see also
Chemical emissions from human activity
Human activities, particularly those involving combustion, contribute significantly to air pollution. The combustion of any carbon-containing fuel releases a range of pollutants, including carbon dioxide (CO2), heavy metals, sulfur dioxide (SO2), nitrogen oxides (NOx), and products of incomplete combustion (PICs) such as carbon monoxide (CO), soot particles, and hydrocarbons.
Vehicle emissions are a significant source of air pollution, with cars, trucks, and other vehicles emitting NOx, volatile organic compounds (VOCs), carbon monoxide, and particulate matter. High-temperature combustion in vehicles contributes to ambient air pollution. The transportation sector, including traffic and vehicle emissions, is a primary source of outdoor air pollution, along with industrial activities, power plants, construction sites, waste burning, and fires.
Industrial processes and power generation also release harmful chemicals and gases into the atmosphere. This includes emissions from manufacturing, refineries, industrial boilers, and fossil fuel power plants, particularly coal-fired power stations. The combustion of fossil fuels, such as coal, oil, and natural gas, releases pollutants and greenhouse gases, contributing to climate change. Carbon dioxide, the primary greenhouse gas, accounted for about 79% of the country's total greenhouse gas emissions in 2021. Methane, a powerful greenhouse gas, made up over 11% of emissions and is released during oil and gas drilling.
Household activities, such as cooking, heating, and lighting, also contribute to air pollution. The use of dirty technologies, such as open fires and inefficient stoves, as well as fuels like kerosene, wood, and coal, can emit harmful pollutants indoors. Boiling water for bathing and cooking animal fodder can further add to household air pollution exposures. Additionally, indoor wood-burning has been linked to an increased risk of lung cancer, especially in women.
Agricultural practices can also compromise air quality, with large-scale animal feeding operations emitting pollutants like ammonia gas, which can cause acute lung function problems in children with asthma.
Air Lead Levels in Polluted Cities: A Concern?
You may want to see also
Combustion of carbon-containing fuels
The combustion of carbon-containing fuels has been a source of energy for humanity since the discovery of fire. However, it is also a significant contributor to air pollution. The combustion of carbon-containing fuels releases a range of pollutants into the atmosphere, including carbon dioxide (CO2), carbon monoxide (CO), nitrogen oxides (NOx), and particulate matter such as fly ash and bottom ash.
Carbon dioxide (CO2) is a major combustion product of carbon-containing fuels. While it is not directly harmful to humans, it is a well-known greenhouse gas that contributes to climate change. The combustion of fossil fuels, such as coal, oil, and natural gas, releases large amounts of CO2 into the atmosphere, leading to an increase in atmospheric CO2 concentrations and global warming.
Carbon monoxide (CO) is another important combustion product that can have significant health impacts. It is formed during incomplete combustion when there is insufficient oxygen to completely burn the fuel. Carbon monoxide is a toxic gas that can cause headaches, dizziness, vomiting, and nausea. High levels of carbon monoxide can lead to unconsciousness or even death. Long-term exposure to moderate and high levels of carbon monoxide is also associated with an increased risk of heart disease.
Nitrogen oxides (NOx) are formed during combustion at high temperatures. While nitrogen does not typically take part in combustion, it is converted to NOx at high temperatures. Nitrogen oxides contribute to the formation of ground-level ozone, which is a major component of smog. Ozone is considered a direct pollutant and is known to pose environmental and health risks.
In addition to these primary pollutants, there are also secondary pollutants that are formed in the atmosphere through the reactions of primary pollutants. Fly ash and bottom ash are also considered pollutants, as they can contain heavy metals and other toxic substances.
To mitigate the air pollution caused by the combustion of carbon-containing fuels, various control measures have been implemented. These include pollution control technologies such as scrubbers installed in industrial smokestacks to remove pollutants from exhaust gases. Additionally, the development of alternative fuels and the improvement of combustion processes to reduce the formation of pollutants are also being explored.
Air Pollution: Cities with the Dirtiest Air Globally
You may want to see also
Machine learning and air quality data
Machine learning has emerged as a powerful tool for predicting and monitoring air quality, with the potential to save millions of lives globally. The increasing air pollution crisis poses a significant threat to human health, ecosystems, and the earth's climate. Traditional methods of forecasting air quality have employed statistical methods like ARIMA to predict the levels of air pollutants. However, with the increasing availability of historical data, machine learning models have gained prominence as they can provide more accurate forecasts.
Machine learning algorithms can utilise large datasets with various parameters and measurements to predict air quality. These models can be trained on data from different cities worldwide, incorporating information on air pollutants such as carbon monoxide (CO), ozone (O3), nitrogen dioxide (NO2), and particulate matter (PM2.5). The data preprocessing step is crucial for the performance of machine learning algorithms, involving handling missing data, removing outliers, data transformation, and feature engineering.
Several machine learning models have been applied to air quality prediction, including Random Forest, Logistic Regression, Decision Tree, Support Vector Machine (SVM), Linear SVC, K-Nearest Neighbor, and Multinomial Naïve Bayes. These models can provide real-time alerts on air quality status, helping to prevent health issues associated with air pollution, such as respiratory and cardiovascular problems. For instance, the AirNet model employs advanced machine learning techniques to predict air quality and provide valuable insights for decision-makers to mitigate contamination.
Furthermore, specific machine learning approaches, such as support vector regression (SVR), have been proposed for hourly air quality forecasting in regions like California. SVR aims to build models for each pollutant and particulate measurement, as well as the overall hourly air quality index. Optimising SVR model performance involves selecting appropriate kernel functions and penalty parameters, and comparing its performance with other machine learning algorithms.
Overall, machine learning offers a transformative approach to combat air pollution and its adverse effects. By harnessing large datasets and advanced algorithms, machine learning models can provide early detection of air quality issues, support decision-making, and ultimately protect public health and the environment.
London's Dark Days: Air Pollution's Deadly History
You may want to see also
Frequently asked questions
A mass balance is a calculation that considers the input and output of a system to determine how mass is distributed within it.
Mass balance can be used to model the dispersion of pollutants in the air and predict their concentrations. This is particularly important for indoor air quality, which can be worse than outdoor air quality and negatively impact human health and productivity.
One example is predicting the concentration of CO2, a common indoor air pollutant, in a building with interconnected rooms using a multiple compartment mass balance model. Another example is predicting real-time within-vehicle air pollution exposure using mass balance and machine learning approaches.
The inputs for a mass balance calculation of air pollution can vary depending on the specific application but may include factors such as pollutant emissions, meteorological data, and chemical transformation mechanisms or ventilation conditions.
Some common tools or models used in mass balance calculations for air pollution include receptor models such as the Chemical Mass Balance (CMB) model developed by the EPA, principal component analysis (PCA), positive matrix factorization (PMF), and machine learning algorithms.