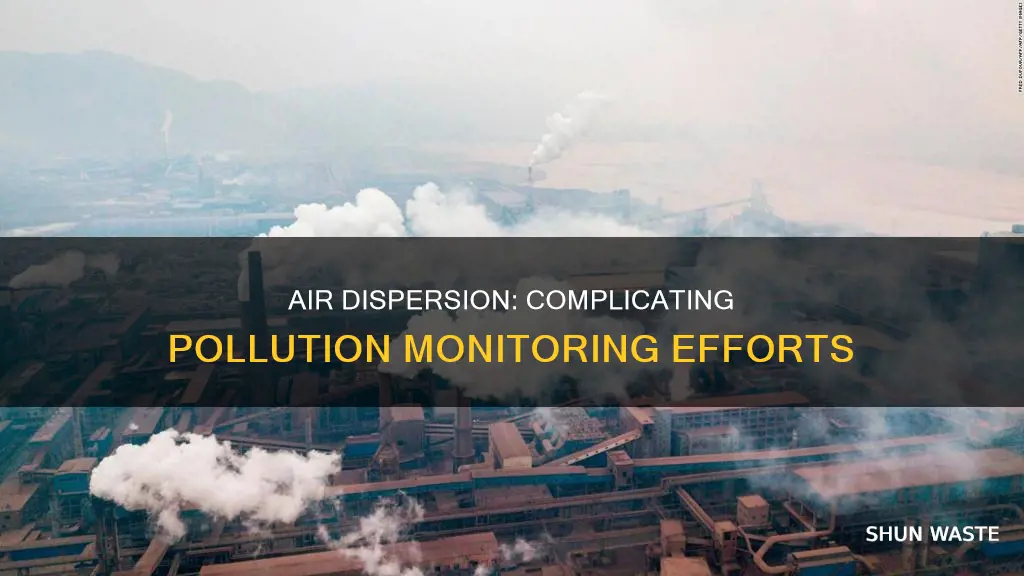
Air dispersion is a complex process influenced by various factors, including wind speed and direction, topography, meteorological conditions, and emission sources. This complexity poses significant challenges to air pollution monitoring and modelling. The dispersion of air pollutants can occur via air, water, soil, living organisms, and food, with varying pathways and rates depending on the emission source and pollutant type. Air pollution dispersion modelling plays a critical role in understanding and mitigating the impacts of air pollution on public health, the environment, and climate. However, the development and implementation of accurate models require substantial resources, skilled personnel, and advanced technology. Insufficient monitoring stations and data sparsity further complicate the task of accurately assessing air quality and predicting pollution dispersion patterns.
Characteristics | Values |
---|---|
Modelling air pollution dispersion | Dispersion models are used to predict future air pollution concentrations and are important for governmental agencies tasked with managing air quality. |
Data requirements | Developing accurate models requires vast amounts of data on emission sources, meteorological conditions, topography, and atmospheric chemistry. |
Data limitations | Reliable data is often limited, especially in certain regions with insufficient monitoring stations, which can lead to inaccurate predictions and hinder the development of effective pollution control strategies. |
Temporal variations | Air pollution levels exhibit temporal variations, including long-term trends, annual variations, and marked seasonal, weekly, and daily patterns. Measurements of exposure will vary depending on when, where, and for how long monitoring is conducted. |
Dispersion pathways | Air pollutants may be dispersed via air, water, soil, living organisms, and food. The pathways depend on the emission source, the pollutant type, and environmental conditions such as wind speed, wind direction, and atmospheric stability. |
Dispersion direction | Dispersion occurs in both vertical and horizontal directions. Horizontal dispersion is influenced by wind speed, direction, and topography, while vertical dispersion is influenced by thermodynamics ("warm air rises"). |
Mixing | The mixing layer in the Earth's atmosphere, located between the surface and the inversion layer, is where most airborne pollutants are transported and dispersed. Mixing is influenced by wind speed, temperature inversions, and the presence of moving vehicles. |
Unique challenges | Urban areas pose unique challenges due to the complex nature of wind flows relative to building geometries, the variety of pollution sources, and the high population density. |
What You'll Learn
Mathematical modelling
At its core, air dispersion modelling aims to characterise the complex atmospheric processes that govern how pollutants disperse. By accounting for various factors, such as emission sources, meteorological conditions, and atmospheric layers, these models can predict the concentration of pollutants at specific locations and under different scenarios. This predictive capability is invaluable for air quality management and policy-making.
One of the key advantages of air dispersion modelling is its ability to assess multiple sources and locations within short timeframes, ranging from hours to days. This efficiency contrasts with traditional air monitoring, which can take months or even years to collect data from a single location. By expediting the data collection process, air dispersion modelling enables more timely decision-making and the ability to address urgent concerns, such as emergency planning for accidental chemical releases.
The versatility of air dispersion modelling is evident in its applications. These models can be used to identify emission sources, evaluate the effectiveness of regulatory measures, and predict future pollutant concentrations under changing conditions. For example, they can help determine whether industrial facilities comply with National Ambient Air Quality Standards (NAAQS) and other regulatory requirements, such as New Source Review (NSR) and Prevention of Significant Deterioration (PSD) regulations.
Furthermore, air dispersion modelling offers a more nuanced understanding of pollutant dispersion by considering factors like stack height, diameter, velocity, temperature, and mass pollutant emission rates. By accounting for these variables, models can more accurately define abatement requirements and stack heights, optimising designs to minimise pollution and costs. This level of detail is often lacking in basic assessment methods.
Breathing Easy: Where to Find the Purest Air
You may want to see also
Meteorological conditions
Wind speed and direction are key factors in the dispersion of air pollution. Horizontal dispersion, or how far pollution spreads at a given atmospheric level, is primarily driven by wind speed and direction. For instance, a faster wind speed will result in a more elongated pollution plume, reaching a greater distance. The topography of an area can also influence horizontal dispersion. Pollution may become trapped in certain areas, such as in the entrance of a valley or in a city surrounded by mountains, if the wind speed is insufficient to carry it over these barriers.
Vertical dispersion, on the other hand, is influenced by thermodynamics. Warmer air rises, and this principle impacts the dispersion of pollutants. The temperature profile of the atmosphere plays a role in determining how pollution disperses vertically. Additionally, the turbulent dynamics of wind are strongest at the Earth's surface, and the part of the planetary boundary layer (PBL) between the Earth's surface and the bottom of the inversion layer is known as the mixing layer, where most airborne pollutants are transported and dispersed.
Dispersion modelling techniques, such as the Gaussian model, Lagrangian model, and advanced models like AERMOD and ADMS 4, incorporate meteorological inputs to predict pollutant concentrations at selected downwind locations. These models assist in ensuring compliance with air quality standards, designing control strategies, and informing emergency planning for accidental chemical releases.
Beijing's Air Pollution: A Hazardous Health Crisis
You may want to see also
Environmental conditions
The emission height is another crucial factor. Ground-level sources like road traffic emit pollutants closer to the surface, while tall chimneys or industrial plants release pollutants from higher altitudes. The interaction of these emissions with the varying wind conditions at different heights influences the dispersion outcomes. Additionally, the emission source itself matters—a fixed point source, such as a chimney, differs from a diffuse source like multiple vehicles or solvents.
The complexity of environmental conditions and their impact on dispersion patterns makes modelling and measuring pollutant behaviour challenging. This complexity is further exacerbated by the uniqueness of each situation, especially in urban areas with intricate geometries, like street canyons. The intricate interplay of environmental factors leads to temporal and spatial variations in pollution levels. For instance, long-term trends may reflect economic shifts or policy interventions, while annual variations can be attributed to inter-year climate differences. Moreover, many pollutants exhibit marked seasonal, weekly, and daily patterns due to activity cycles and short-term climatic fluctuations.
The impact of environmental conditions on air pollution dispersion is a dynamic and multifaceted process. It involves the interaction of meteorological factors, emission characteristics, and the physical behaviour of pollutants themselves. This complexity underscores the challenges faced in air pollution monitoring and the need for sophisticated modelling techniques, reliable data, and sufficient resources to effectively understand and mitigate the adverse effects of air pollution.
Waste Incineration: Air Pollution or Clean Energy?
You may want to see also
Monitoring infrastructure
Monitoring air pollution is a complex task that requires a comprehensive infrastructure. This infrastructure includes monitoring stations, advanced modelling programs, and skilled personnel. However, in certain regions, the number of monitoring stations is insufficient, leading to sparse data points. This challenge is evident in India, where the existing air quality monitoring infrastructure is limited, hindering the validation and accuracy of dispersion models.
The complexity of air dispersion makes it difficult to model or measure pollutant patterns and trends, which are crucial for predicting human exposure levels. Pollutants disperse through various pathways, including air, water, soil, living organisms, and food. The rates and patterns of dispersion depend on factors such as meteorological conditions, emission height, and the source of pollution. For example, ground-level sources like road traffic differ from high-level sources like tall chimneys.
To address these complexities, atmospheric dispersion modelling is employed. This technique uses mathematical simulations to predict how air pollutants disperse in the atmosphere. These models consider meteorological inputs and emissions data to estimate the downwind concentration of pollutants. They are valuable tools for predicting future concentrations, assessing compliance with air quality standards, and designing control strategies to reduce harmful emissions.
The effectiveness of dispersion models relies on the availability of reliable and extensive data. This includes information about emission sources, meteorological conditions, topography, and atmospheric chemistry. However, obtaining accurate data can be challenging, especially in dynamic urban environments with unique pollution sources. The lack of reliable data can impact the accuracy of models and hinder the development of effective pollution control strategies.
To overcome data limitations, organizations such as the Central Pollution Control Board (CPCB) and State Pollution Control Boards in India are responsible for enhancing air quality monitoring and modelling capabilities. These efforts include promoting cleaner technologies, regulating emissions, encouraging public transportation, and implementing dust control measures. By addressing data gaps and improving monitoring infrastructure, more accurate dispersion models can be developed, leading to better-informed decisions for mitigating the adverse effects of air pollution.
Air Quality Standards: National Ambient Air Guidelines Explained
You may want to see also
Regulatory decisions
In the United States, dispersion models are used to determine compliance with National Ambient Air Quality Standards (NAAQS) and other regulatory requirements such as New Source Review (NSR) and Prevention of Significant Deterioration (PSD) regulations. The EPA's Guideline on Air Quality Models, published in 1978 and periodically revised, provides a framework for consistent and equitable use of modelling in the US air quality management system. The models listed in Appendix W are required for State Implementation Plan (SIP) revisions for existing sources and NSR and PSD programs.
The accuracy of dispersion models depends on the quality and reliability of input data, including emission sources, meteorological conditions, topography, and atmospheric chemistry. Sparse or outdated data can lead to inaccurate predictions, hindering the effectiveness of pollution control strategies and regulatory decisions. For example, in India, limited data on emission sources, meteorological conditions, and pollutant concentrations pose significant challenges to accurate modelling and effective regulatory decision-making.
To improve accuracy, models are continually refined and validated using real-world data. Meteorological data, such as wind speed, direction, and stability, play a crucial role in determining how pollutants disperse. Dispersion models can also incorporate complex terrain and emission height to enhance their predictive capabilities. For instance, the AERMOD modelling system considers planetary boundary layer turbulence structure and scaling concepts, while the CTDMPLUS model is designed for use in all stability conditions for complex terrain.
The regulatory use of dispersion models ensures that air pollution monitoring and management are based on scientific data and predictions. By understanding how pollutants disperse, authorities can make informed decisions to protect public health, the environment, and climate. These models enable the identification of high-risk areas, the implementation of targeted interventions, and the assessment of the effectiveness of regulatory measures.
Air Quality Alert: Cities with Hazardous Air
You may want to see also
Frequently asked questions
Air dispersion refers to the movement of air pollutants in the atmosphere, which can occur in both vertical and horizontal directions. Horizontal dispersion refers to how far pollution spreads at a given level of the atmosphere, while vertical dispersion is influenced by thermodynamics.
Air dispersion complicates air pollution monitoring due to the numerous factors that influence it, including meteorological conditions (such as wind speed and direction, and atmospheric stability), emission height, and the source of the pollution. These factors make it challenging to accurately predict the dispersion patterns and exposure levels of pollutants.
Atmospheric dispersion modelling is a commonly used technique that employs mathematical simulations and computer programs to estimate the concentration and dispersal patterns of air pollutants. These models are essential for governmental agencies in managing air quality and ensuring compliance with standards.
Air pollution dispersion modelling faces challenges such as limited data on emission sources, meteorological conditions, and pollutant concentrations. Sparse data points due to insufficient monitoring stations can hinder the accuracy of predictions. Additionally, developing and maintaining advanced dispersion models require significant resources, including skilled personnel, computational power, and continuous data inputs.